Overview
Recent breakthroughs in machine learning (ML) for molecules have demonstrated impressive successes, ranging from highly accurate protein structure prediction to assist the discovery of novel drug candidates and chemical synthesis planning. These achievements position molecular machine learning as a key tool for addressing pressing challenges related to drug and materials discovery.
Course Description & Learning Outcomes
However, current machine learning - especially deep learning (DL) - methods, still face significant challenges and limitations. DL models are (I) data hungry while data in the molecular sciences are often rather sparse, (II) struggle with adaptability to changing tasks or distributions, (III) lack of (properly) incorporating domain knowledge, (IV) missing explainability and inherent differentiation of causation from correlation. Additionally, given the flood of new published methods, thorough benchmarks as well as regulations for deployments and sustainability are needed. This workshop aims to address the current limitations and capabilities of machine learning methods for molecules by (i) critically assessing them both theoretically and in applied and industrial settings, and (ii) showcasing novel and promising approaches to accelerate molecule discovery. We also explore how recent advancements in Large Language Models (LLMs) may revolutionize the field.
Pre-course instructions
Sign up for the event on eventbrite, the necessary links will be sent to participants of the event closer to the date of the event.
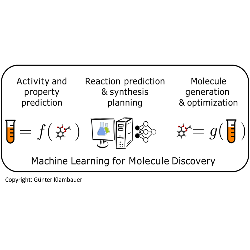
Schedule
Date: 08 Dec 2023, Friday
Time: 9:00 AM - 6:00 PM (GMT +1:00) Brussels, Copenhagen, Madrid, Paris
Location: Online
Pricing
Course fees: $0